What Does Transparency Really Mean in the Context of AI Governance?
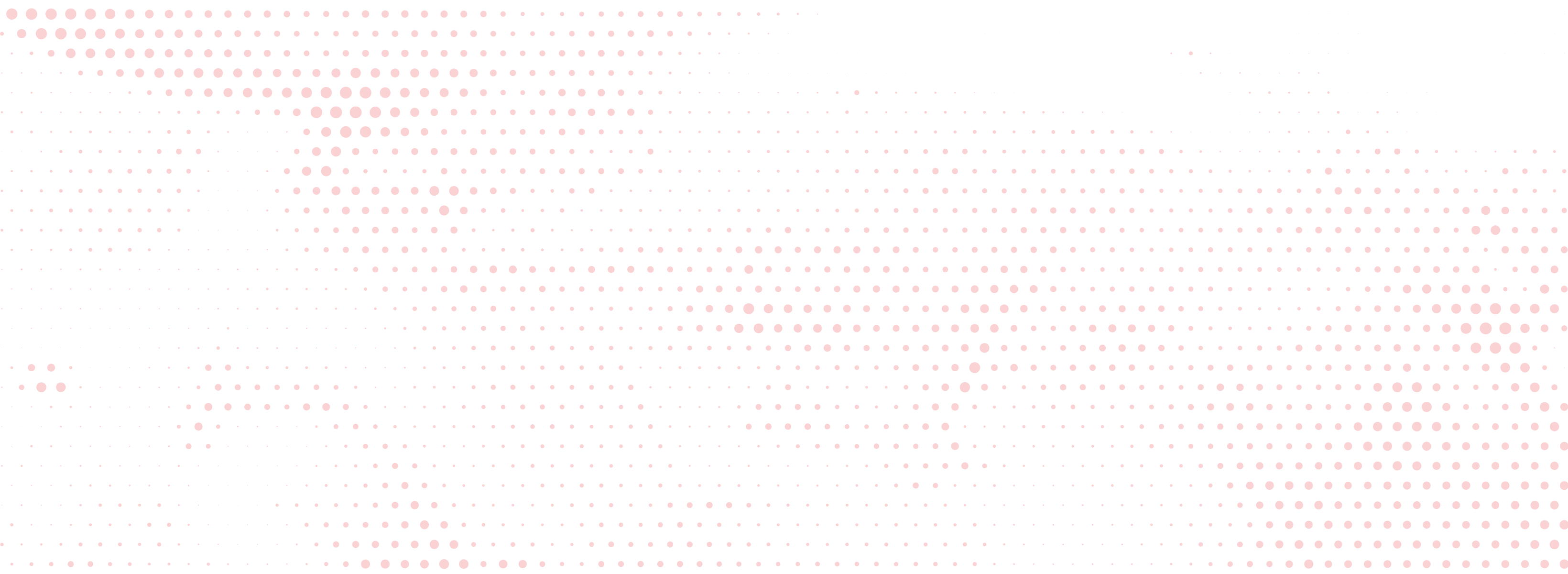
This blog talks about how transparency in AI governance encompasses multiple crucial aspects including model explainability, data transparency, documentation, risk disclosure, bias assessments, governance frameworks, and stakeholder communication - all essential elements for building trust, ensuring accountability, and maintaining ethical AI practices in organizations.
The Multifaceted Challenge of Transparency in AI
Transparency involves clarity and openness about how AI models function, make decisions, and affect users and stakeholders. Leaders must ensure that stakeholders - including users, regulators, internal teams, customers, suppliers, and other affected stakeholders - have access to relevant, understandable information that builds and maintains confidence in the AI applications and results. This is not a “nice-to-have” attribute. It is essential, especially in the early days of AI experimentation and adoption.
Transparency in AI is foundational to building trust, ensuring accountability, and fostering a culture of ethical technology use. It requires organizations to open the “black box” of AI, enabling stakeholders to understand, assess, and hold AI systems accountable. However, achieving transparency is not straightforward; it involves revealing complex, sometimes proprietary processes in a way that is accessible and meaningful to a broad audience. When done right, transparency in AI governance allows for responsible, compliant, and resilient systems. Without it, organizations risk reputational harm, regulatory penalties, and loss of stakeholder trust.
Why Transparency Matters: Real-World Consequences of Opaqueness
The stakes of transparency are high, especially when AI systems are used in critical areas. Here are two examples illustrating what can go wrong when transparency is overlooked:
- Bias in Hiring Models: A large tech company implemented an AI-driven hiring tool that, unbeknownst to users, systematically disadvantaged women. Without transparency around the algorithm’s scoring criteria, the bias persisted for years, harming the company’s reputation and betraying its commitment to diversity.
- Healthcare Decision-Making: A hospital used an AI model to prioritize patient treatments, but when questioned, they couldn’t explain why some high-risk patients were deprioritized. This lack of transparency led to public backlash, undermined patient trust, and damaged the healthcare provider’s standing.
These stories underscore the importance of transparency. When stakeholders don’t understand how decisions are made and or can’t verify that they are fair, trust is eroded, and accountability falters. Transparency isn’t just good practice; it’s necessary for sustainable AI governance.
Key Transparency Elements and Actionable Guidance
Transparency is not about just one thing. It is an umbrella term that covers multiple aspects of AI governance. Below, I outline the critical components of transparency, explaining why each is important, the benefits of addressing it, and actionable steps for leaders to foster transparency effectively.
Explainability of AI Models
Leaders should prioritize explainability in AI models, embedding it throughout the model design, testing, and deployment phases. However, achieving robust explainability is not always straightforward; leaders must recognize that explainability methods and tools are still evolving, with active research seeking to address the limitations of current approaches.
Explainability is fundamental to successful AI adoption, managing risks, and ensuring regulatory compliance. It is especially crucial in high-stakes fields like government, finance, and healthcare, where trust in AI’s fairness and reliability is paramount. Explainability helps to mitigate the “black box” problem, where users lack visibility into how AI arrives at conclusions, potentially leading to misunderstandings, misuse, or outright distrust. By addressing explainability early, and continually, leaders can build stronger, more accountable AI systems that meet stakeholder expectations.
The benefits of explainable AI applications include increased trust and accountability as well as enhanced decision-making. Providing clear explanations promotes confidence in AI outputs, accelerates adoption, and gives stakeholders a basis for holding systems accountable. Trust is more likely to build when users understand how AI arrives at critical decisions.
Transparent AI systems enable users and stakeholders to make informed choices, as they can better understand the underlying logic and assess the implications of AI-driven recommendations.
A Realistic Perspective on ExplainabilityAI explainability is inherently challenging, particularly with complex models like deep learning networks, which often rely on vast numbers of parameters and intricate internal representations. Many AI models were not initially designed with explainability in mind, making it difficult to “retrofit” transparency into them. Current explainability techniques—such as SHAP (Shapley Additive Explanations) or LIME (Local Interpretable Model-agnostic Explanations)—offer insights but often fall short of providing complete transparency, particularly in high-dimensional or adaptive models. Additionally, explainability efforts can sometimes impact model performance, leading to trade-offs between transparency and accuracy.
Explainability needs vary across different sectors, use cases, and stakeholders. What’s sufficient for a data scientist may be too technical for a regulator or a non-technical business leader. Striking the right balance between detail and accessibility is crucial and adds to the complexity of explainability in AI.
Guidance for Leaders in Navigating Explainability ChallengesGiven these complexities, leaders should stay informed of advancements in explainability methods and invest in both technical and non-technical tools to facilitate transparency. Here are some key strategies for keeping ahead:
Actionable Steps:
- Stay Updated on Research and Tools: Explainability is a fast-moving area of AI research. Encourage teams to stay informed of emerging techniques, like counterfactual explanations or hybrid models that inherently support interpretability. Regularly assess new tools to determine their applicability in your organization.
- Choose Transparent Models When Possible: For certain applications, simpler or inherently interpretable models (e.g., decision trees or rule-based systems) may meet the needs for explainability without sacrificing too much performance. When accuracy and complexity are necessary, supplement complex models with post-hoc explainability techniques that help translate insights for stakeholders.
- Model Logic and Decisions: Adopt explainable AI (XAI) methods that provide insight into decision-making, focusing on high-stakes use cases where transparency is critical. Collaborate with technical teams to select or design models with inherent transparency whenever feasible, as opposed to relying solely on post-hoc explanations.
- User-Friendly Explanations: Invest in resources to communicate complex model logic in accessible terms. Developing user interfaces or dashboards that translate explanations for non-technical stakeholders can bridge the gap between technical transparency and practical understanding.
- Conduct Stakeholder-Specific Explainability Testing: Implement testing for explainability that aligns with different stakeholder needs. Run sessions with end-users, regulators, and business leaders to ensure the explanations provided meet their specific requirements and expectations.
Future-Proofing Explainability EffortsExplainability will likely become increasingly expected in both ethical standards and regulations. Leaders should consider explainability as an evolving objective and treat it as a core competency within the organization. By cultivating expertise and building processes that support explainability from design to deployment, organizations can better manage emerging expectations, boost AI adoption, and ensure that AI systems remain trustworthy and accountable as technologies advance.
Data Transparency
Leaders should ensure that stakeholders are fully informed about data origins, lineage, quality, and privacy practices, as data transparency forms a core component of effective AI governance. As a foundational element of AI systems, transparency around data sources, accuracy, and potential biases directly influences model reliability and fairness. Pursuing data transparency is not only ethically sound but also aligns with legal and regulatory requirements, fostering trust and preventing the propagation of hidden biases.
Data transparency is an outcome to aim for in any robust data governance program, which should ensure high-quality data collection, ethical handling, and clear accountability. Transparent data practices give stakeholders visibility into how AI systems are built and enable informed trust in the systems' outcomes. Inconsistent or opaque data can lead to skewed model outputs, reputational harm, and compliance risks, especially as regulatory scrutiny around data use intensifies.
The benefits of data transparency include:
- Better Data Quality and Reliability: Transparency helps validate data sources, making it easier to ensure that data is accurate, representative, and free from hidden biases. Clear insights into data quality prevent downstream issues and reinforce stakeholder trust in AI-driven decisions.
- Regulatory Compliance: Many data privacy laws and regulations require organizations to disclose data handling practices. By adhering to these standards, organizations mitigate risks of non-compliance, reduce exposure to potential fines, and demonstrate a commitment to ethical data use.
For more detailed guidance on building strong data governance practices to support transparency, please refer to Blog #7 in this series, which explores how to address the overall challenges of data governance in AI.
Actionable Steps:
- Data Sources and Quality: Conduct regular audits to assess data source validity and disclose origins to stakeholders where feasible. Track data quality metrics, and implement processes for validating data diversity and fairness, which helps mitigate biases that could influence AI outcomes. Proactive data governance processes, as discussed in Blog #7, are key to building transparency and preventing data-related issues in AI models.
- Data Privacy: Provide transparent data-use statements detailing how personal data is collected, stored, and processed. Make these statements readily accessible to users, partners, and regulatory bodies. Adopting clear data-use policies not only supports compliance but also builds public confidence in the organization’s commitment to responsible AI.
The Role of Data Governance in Achieving TransparencyData transparency is a result of rigorous data governance efforts, which include practices to manage data lineage, enforce data quality standards, and ensure compliance. In Blog #7, we discuss how organizations can adopt structured data governance approaches that address the quality and integrity of data throughout its lifecycle. By focusing on these aspects, leaders can achieve transparency not just as a one-time goal but as an enduring part of the AI development process, ultimately strengthening their data strategy and supporting ethical AI.
Model Governance and Documentation
Robust model documentation ensures AI systems are traceable and that decisions align with policy, governance, and ethical standards. Comprehensive documentation provides a roadmap for responsible AI use, allowing organizations to track model modifications and maintain alignment with governance standards. It also enables internal and external reviews, supporting accountability.
The benefits of model governance and documentation include streamlined sudits and compliance as well as historical insight. Clear documentation facilitates compliance with regulations and provides an accessible reference for audits. Version tracking allows teams to understand the model’s evolution, aiding in diagnosing issues retrospectively.
Actionable Steps:
- Model Documentation: Maintain detailed records of model creation, purpose, testing, and known risks. Ensure this documentation is accessible to stakeholders to enable accountability and alignment with governance goals.
- Version Control and Auditability: Implement version control tools to monitor model changes over time, facilitating transparency in model evolution.
Risk and Impact Disclosure
Leaders must prioritize transparent communication about the risks associated with AI systems, particularly when these systems have far-reaching impacts on individuals, communities, or critical business decisions. A robust approach to risk and impact disclosure goes beyond regulatory compliance—it reflects a deep commitment to responsible AI use and societal accountability.
Transparency in risk and impact disclosure allows stakeholders to understand the potential consequences of AI use, fostering a culture of accountability and enabling proactive risk management. Risks related to AI models—such as alignment issues, biases, or unintended consequences—cannot be contained if they are hidden. When organizations disclose these risks openly, they contribute to a culture of learning that benefits both industry and society, building trust in AI technology. On the contrary, withholding information about risks and impacts can lead to reputational damage, regulatory scrutiny, and loss of stakeholder trust.
Transparency also plays a pivotal role in managing “alignment and containment.” When AI systems misalign with their intended goals or produce undesirable outcomes, honest disclosure is essential to contain harm and guide future improvements. Although acknowledging mistakes or revealing system vulnerabilities can be difficult for some leaders, particularly in cultures where admitting fault is discouraged, a commitment to transparency is essential for AI’s long-term, positive impact on society.
Real-World Case Study: Doing It RightIn 2019, a leading financial services company faced issues with an AI-driven credit scoring system that produced biased outcomes disadvantaging certain demographics. Rather than ignore the problem, the company disclosed the issue, provided a detailed explanation of its root cause, and outlined steps taken to address and prevent such bias in the future. This transparency not only helped restore public trust but also prompted valuable industry-wide discussions on the need for fairness in AI applications. The company’s openness set a standard for responsible risk management and demonstrated the benefits of proactively addressing AI-related risks.
Real-World Case Study: Doing It WrongConversely, in 2020, a high-profile healthcare organization deployed an AI system to manage patient prioritization. Reports surfaced that the system was systematically under-prioritizing minority patients, but rather than address these concerns publicly, the organization initially downplayed the issue, leading to a public outcry when investigative journalists exposed the bias. The lack of transparency resulted in significant reputational damage, regulatory scrutiny, and loss of stakeholder trust. This example underscores the risks of withholding information about AI system impacts, particularly when dealing with vulnerable populations.
The benefits of effective risk and impact disclosure are enhanced trust and safety as well as better risk mitigation. When organizations are transparent about risks, they build user trust, empower stakeholders, and reduce the likelihood of hidden risks causing harm. Transparency allows stakeholders to anticipate potential challenges and respond proactively, leading to more robust and resilient AI deployments.
Actionable Steps:
- Identified Risks: Develop and maintain a comprehensive risk registry that catalogs potential biases, inaccuracies, and security vulnerabilities within AI systems. This registry should be updated regularly and accessible to relevant stakeholders. Include documented risk mitigation steps so that all parties understand how risks are addressed.
- Impact on Stakeholders: Conduct regular stakeholder impact assessments, particularly for AI applications that carry high-stakes consequences in sectors such as healthcare, finance, and criminal justice. Ensure that both positive and negative impacts are documented and communicated to stakeholders, so they can make informed decisions based on the full scope of potential outcomes.
Aligning Organizational Culture with Transparency GoalsFor many organizations, embracing transparency in risk disclosure represents a cultural shift. Leaders can cultivate a culture that values transparency by setting clear expectations, rewarding honest reporting, and encouraging open discussions about AI’s limitations and risks. Rather than focusing on fault, organizations should view the identification of AI system issues as an opportunity for improvement. Embracing this mindset not only supports alignment with ethical AI principles but also strengthens an organization’s resilience and adaptability in the face of evolving challenges.
Looking Forward: Risk and Impact Disclosure as a Social ResponsibilityThe societal benefits of AI can only be realized if we openly address the technology’s risks. By sharing knowledge about AI’s real-world impacts—both positive and negative—organizations contribute to a broader understanding of how to safely and effectively deploy these systems. Transparency in risk and impact disclosure is a public good that accelerates learning across industries, informing best practices and shaping responsible AI innovation.
Bias and Fairness Assessments
Leaders must prioritize routine assessments to detect and address biases in AI systems, ensuring they operate fairly, align with ethical standards, and mitigate discriminatory impacts. Bias assessments should be built into every stage of the AI lifecycle—from initial model training to pre-deployment testing and ongoing post-deployment monitoring.
Bias in AI models has become a primary focus in AI laws, rules, and regulations, second only to data privacy, and will be an area of intense scrutiny from regulators. The risks extend beyond regulatory compliance; bias in AI can severely harm an organization’s reputation if exposed, eroding stakeholder trust and damaging brand equity. Proactively conducting bias assessments signals to stakeholders that the organization is committed to ethical and fair AI practices. Furthermore, developing a clear, structured response program for addressing findings of bias or ethical concerns is crucial for minimizing harm if bias is detected.
The stakes of AI bias are high. Bias in models used for hiring, credit scoring, or healthcare can lead to discriminatory outcomes, harming individuals and exacerbating inequalities. Leaders must recognize that failure to address bias proactively not only opens the organization to legal and regulatory repercussions but also impacts societal trust in AI—a foundational element for any organization seeking to build or maintain a reputation for responsible innovation.
The benefits of routine bias and fairness assessments include:
- Improved Fairness and Inclusivity: Identifying and addressing biases in AI enhances the inclusivity and fairness of AI outputs, fostering a reputation for ethical and equitable practices.
- Regulatory Preparedness: Many current and emerging regulations will require companies to demonstrate bias mitigation measures. Routine bias assessments help organizations meet these standards, reducing the risk of non-compliance.
- Reputational Protection: By implementing bias assessments as part of their standard AI governance practices, organizations are better prepared to respond if issues arise, protecting their reputation and demonstrating a proactive stance on ethical AI.
Technology and Tools to Support Ongoing Bias DetectionModern AI tools and technologies offer powerful methods for detecting bias, even in highly complex models. Explainability techniques, statistical audits, and fairness metrics can reveal bias in a model’s predictions, while advanced AI tools can monitor bias on an ongoing basis. By leveraging “AI on AI” tools, organizations can automate bias detection and establish alerting functions that notify the appropriate people of emerging risks. This capability allows organizations to course-correct in near real-time if fairness metrics start to drift or if an unanticipated bias arises.
For example, tools like IBM’s AI Fairness 360 and Microsoft’s Fairlearn provide frameworks for identifying and mitigating bias, while platforms like H2O.ai and DataRobot offer built-in fairness metrics that support proactive bias monitoring. These tools are particularly useful for complex AI models, where it can be challenging to pinpoint sources of bias manually. Leaders should be aware that the capabilities for bias detection are advancing quickly, and staying informed on available technologies will allow them to continuously strengthen their bias assessment programs.
Actionable Steps:
- Bias Detection and Mitigation: Perform bias assessments routinely, using available tools and frameworks to identify and address biases in training data, model logic, and outcomes. During these audits, document findings and actions taken to mitigate bias, and make summaries available to relevant stakeholders. Automated bias detection can provide early warnings and help prevent biases from becoming systemic issues.
- Bias Reporting: Establish a formal bias reporting system that regularly updates stakeholders, regulators, and the public on fairness metrics and actions taken to maintain model integrity. Providing transparency around bias metrics and mitigation steps builds credibility and demonstrates accountability.
Building a Culture of Accountability in Bias AssessmentBias assessments are not a one-time task but an ongoing responsibility. To make bias detection truly effective, organizations should cultivate a culture where bias is openly addressed, and stakeholders are empowered to question model fairness. Leaders should foster cross-functional collaboration among data scientists, ethicists, and compliance teams to ensure a holistic approach to fairness. Bias assessments should be seen not as a compliance obligation but as a commitment to upholding the organization’s values.
Looking Forward: Bias Assessment as an Ethical and Strategic ImperativeIn an environment where AI technology is rapidly evolving, bias assessments are essential to both ethical AI use and long-term strategic success. With AI systems increasingly influencing areas of public trust, like healthcare, employment, and finance, organizations have a responsibility to pursue fairness diligently. By implementing routine assessments and adopting the latest AI-powered tools for bias detection, organizations can stay ahead of regulatory requirements and position themselves as leaders in ethical AI. Furthermore, transparent and proactive bias assessment programs reinforce AI governance frameworks and ensure that AI serves to benefit all, rather than perpetuate existing inequities.
Governance Framework Transparency
Leaders should clearly communicate AI governance frameworks to foster trust in organizational decision-making and accountability. By transparently sharing governance policies, roles, and ethical standards, organizations can build stakeholder confidence and ensure that AI systems are managed responsibly.
Transparency in AI governance frameworks is one of the most powerful ways for organizations to establish credibility, promote trustworthiness, and create a foundation for responsible AI growth. An open governance framework allows stakeholders—both internal and external—to see how decisions are made, who is responsible, and what ethical guidelines are in place. This clarity promotes 360-degree feedback, enabling continuous improvement of AI governance practices and fostering a sense of collective responsibility across all levels of the organization.
For internal stakeholders, knowing the framework and understanding their specific roles within it provides direction and accountability, reducing ambiguity and ensuring alignment with organizational goals. Externally, demonstrating a transparent governance framework shows regulators, customers, and the public that the organization is committed to responsible AI. If regulatory scrutiny occurs, a transparent governance approach reassures authorities that the organization has implemented robust, thoughtful measures to manage AI-related risks effectively.
The benefits of being transparent about AI governance frameworks are:
- Clear Accountability: Transparency in governance frameworks ensures that stakeholders know whom to contact for questions or concerns, building accountability at every level. This clarity supports a proactive approach to managing AI-related risks and enables rapid responses to emerging issues.
- Better Internal Alignment: A defined governance framework fosters team cohesion and consistency in practices. When roles and responsibilities are clearly outlined, departments can work together more effectively, creating a culture of accountability and shared responsibility.
- Enhanced Trustworthiness and Regulatory Readiness: In an era of increasing regulatory scrutiny, a transparent governance framework shows regulators that the organization is prepared and committed to compliance. It provides clear evidence of how AI is managed, monitored, and ethically guided, demonstrating a proactive stance on risk management.
Aligning with Regulatory ExpectationsThere is much to be gained by being public about AI Governance programs, especially as it pertains to regulatory authorities. Just as one example, take note of the recent updates to the U.S. Department of Justice (DOJ) guidance on the Evaluation of Corporate Compliance Programs and how they underscore the importance of AI governance transparency. The DOJ now considers questions such as:
- How does the company assess the impact of new technologies, like AI, on its ability to comply with laws?
- Is risk management of AI integrated into broader enterprise risk management strategies?
- How does the company curb potential unintended consequences or misuse of AI?
- Does the company have controls to monitor and ensure AI’s trustworthiness, reliability, and compliance with law and company policies?
- How does the company hold employees accountable for AI use?
These questions illustrate an increasing interest in how organizations govern AI, manage risks, and prevent misuse. By addressing these points through a clear, transparent governance framework, companies are better prepared to answer regulatory questions, demonstrate ethical compliance, and showcase accountability mechanisms.
Actionable Steps:
- AI Policies and Frameworks: Share your organization’s governance framework with both internal and external stakeholders. Internally, ensure that employees understand the AI policies guiding their work, and externally, consider publishing a simplified version of the framework to build trust with customers, partners, and regulators. This approach aligns with DOJ’s recommendations to establish clear policies for AI’s use, risk management, and oversight.
- Roles and Responsibilities: Clearly define and communicate who oversees key governance tasks, including model management, risk review, and ethics oversight. Ensure that all employees understand their role within the governance framework, from data scientists to compliance officers. Consider creating a designated AI ethics committee or governance team to address specific risks, evaluate ethical concerns, and maintain accountability.
Encouraging a Culture of Transparency and AccountabilityAn effective governance framework does more than establish rules; it encourages a culture of transparency and accountability across the organization. Leaders should promote open discussions about AI risks, ethical considerations, and governance practices, making it clear that raising concerns or sharing feedback is not only encouraged but valued. This cultural shift supports both the organization’s AI governance and its broader commitment to ethical, responsible innovation.
Achieving Continuous Improvement through Stakeholder FeedbackSharing governance frameworks with internal and external stakeholders provides valuable opportunities for feedback. By regularly revisiting and refining governance practices based on input from employees, customers, and regulators, organizations can stay ahead of emerging AI challenges. When frameworks are transparent and accessible, they enable a two-way dialogue that drives continuous improvement.
Transparency in Governance Frameworks as a Strategic AdvantageIn a world where AI regulations are rapidly evolving, governance framework transparency is no longer optional; it’s a strategic necessity. Transparency demonstrates to stakeholders that the organization is proactive, ethical, and prepared to adapt to new challenges. By embracing a transparent governance framework, leaders can enhance their organization’s reputation, build resilience, and establish a competitive advantage based on trust and accountability.
Stakeholder Communication
Regular, open communication with stakeholders is essential for building understanding, managing expectations, and establishing trust. Effective communication helps stakeholders understand AI’s capabilities and limitations, reduces misunderstandings, and provides a mechanism for feedback and engagement.
This section reinforces and extends what we have already discussed above, especially in the sections on “Risk and Impact Disclosure” and “Governance Framework Transparency.”
The benefits of effective stakeholder communication are:
- Increased Trust: Transparency fosters confidence in the organization’s commitment to responsible and ethical use of AI.
- Enhanced User Experience: Informed stakeholders make better decisions and feel respected.
Actionable Steps:
- Regulatory Reporting: Create a roadmap for meeting transparency requirements in regulatory standards. Prepare protocols to disclose AI use, risks, and compliance to regulatory authorities.
- User and Customer Communication: Offer clear explanations about AI’s functionality, data use, and decision processes. Enable feedback channels to address questions or concerns.
By integrating transparency into AI governance, organizations can build systems that are trustworthy, resilient, and ethically sound, demonstrating to stakeholders that AI is used responsibly and with their interests in mind.
Featured in: AI / Artificial Intelligence